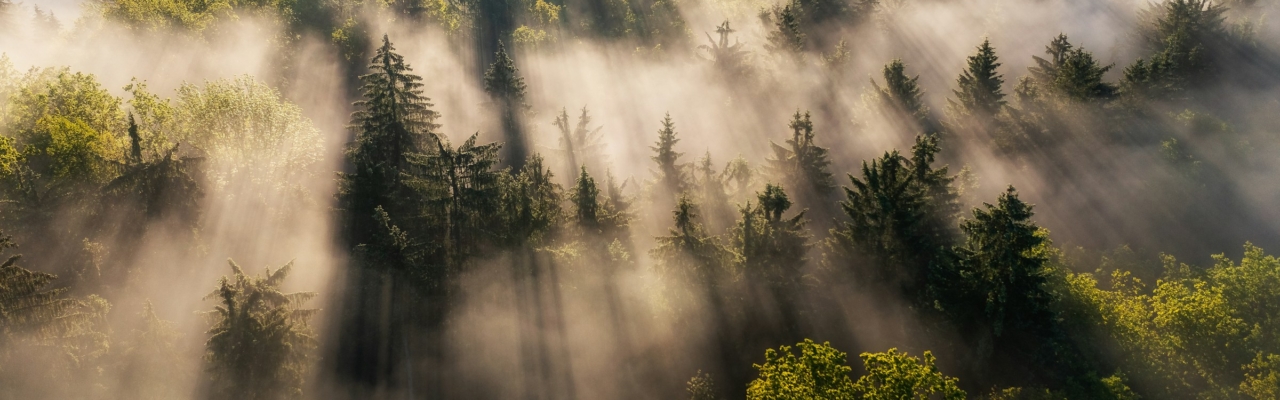
Earth System Models are the main tools scientists use to study and improve our understanding of the climate system and project climate change. However, even the best models still exhibit uncertainties in their projections. New research led by CMCC introduces a novel method that reduces uncertainties in near-surface air temperature projections by up to 54% compared to state-of-the-art approaches, making predictions more precise and reliable. This can help provide better guidance for governments and communities in planning effective adaptation and mitigation strategies to meet the challenges posed by climate change.
Since the Industrial Revolution, anthropogenic activities have caused a dramatic increase in the atmospheric concentration of CO2 and other greenhouse gases, reaching unprecedented levels that have led to a warming planet. The latest report from the Copernicus Climate Change Service shows that 2024 was the hottest year on record since 1850, also marking the first time the global temperature exceeded 1.5°C above pre-industrial levels – a critical threshold set by the Paris Agreement to reduce the risks and impacts of climate change.
Climate science plays a key role in improving our understanding of these impacts and the climate system at large. New research, led by CMCC researcher Francesco Immorlano, in collaboration with Columbia University, University of California Irvine, and Deutsches Zentrum für Luft- und Raumfahrt (DLR) e.V. German Aerospace Center, introduces a novel method that exploits Transfer Learning (TL) to constrain climate model simulations to real observations. This approach reduces uncertainties in near-surface air temperature projections, making them more precise and reliable, thus providing better guidance for governments and communities in planning effective adaptation and mitigation strategies.
“ML not only narrows the uncertainty of long-term temperature projections but also sets a new standard for the use of artificial intelligence to bridge the gap between complex climate models and real-world observations. This also marks a significant step forward in modeling future climate, which has far-reaching implications for assessing and managing climate change risks on a global scale,” says CMCC researcher, Giovanni Aloisio, who supervised the study in collaboration with Pierre Gentine.
The study significantly reduces the uncertainty range in long-term temperature projections (as seen in the figure below) between 2081 to 2098 (relative to the pre-industrial period of 1850 to 1900) under Shared Socioeconomic Pathway (SSP) 2-4.5, by 47% compared to the best state-of-the-art approach (Ribes et al., 2021) and by 54% compared to the estimates provided by IPCC AR6 WG1 (IPCC, 2023), with similar improvements also observed under SSPs 3-7.0 and 5-8.5.
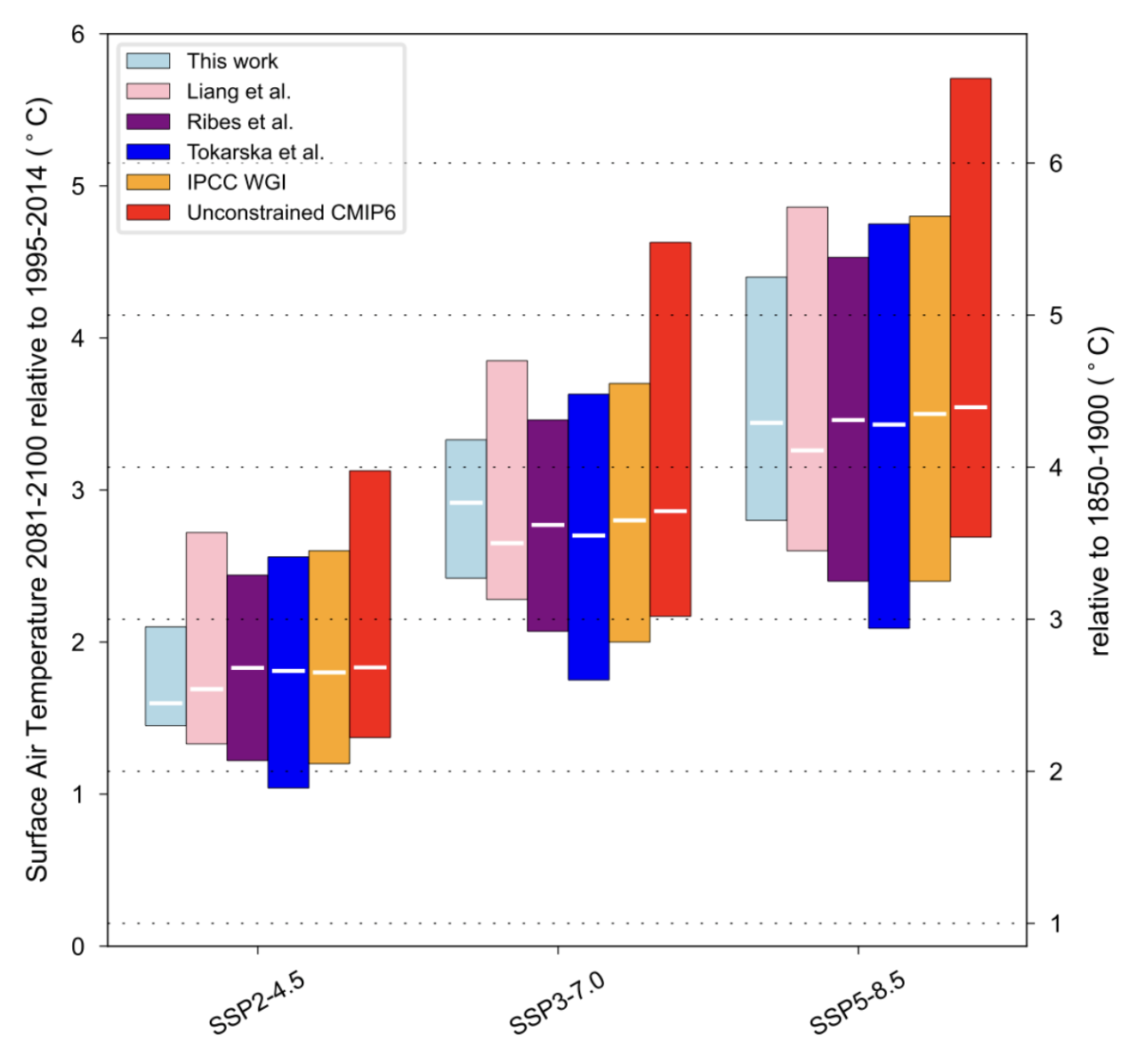
Global surface air temperature changes for the long-term period (2081–2100). Source: Immorlano et al., 2025
“The main innovation in this work lies in using TL to enhance long-term projections of near-surface air temperature maps,” says Immorlano. “This allows us to efficiently combine the strengths of an ensemble of CMIP6 models’ simulations with historical observations spatially resolved on a global scale.”
“This is a powerful example of how ML can be leveraged to reduce uncertainties in long-term climate projections under different SSPs. While significant efforts have been made to adopt these techniques for emulating atmospheric and oceanic systems, until now progress in actually reducing uncertainties remains limited,” says CMCC researcher and co-author of the study Gabriele Accarino. “This challenge is critical, as high uncertainties hinder the ability of policy makers to design precise and effective adaptation and mitigation strategies. This work therefore represents an important step forward, demonstrating the potential of ML to improve the reliability of Earth System Model simulations for decision-making in climate policy.”
“ML is paving the way for new approaches for supporting climate science. This study shows how a novel data-driven method —TL —can be effectively employed to reduce the uncertainty in long-term temperature projections. By integrating historical observations with the knowledge of climate models, it demonstrates the potential of innovative ML solutions in improving climate projections,” says CMCC researcher and co-author of the study Donatello Elia.
TL is a recent cutting-edge technique in Machine Learning (ML) that enables the exploitation of knowledge acquired by a pre-trained model on a data-rich task as a foundation for enhancing performance on a new but related task within the same domain, even with limited data availability. This method reduces uncertainty in projections by ensuring that the Deep Neural Networks (DNNs) learn to replicate the detailed spatial patterns of climate simulations while constraining them to the historical real-world temperature trends.
For more information:
Immorlano, V. Eyring, T. le Monnier de Gouville, G. Accarino, D. Elia, S. Mandt, G. Aloisio, P. Gentine, Transferring climate change physical knowledge, Proc. Natl. Acad. Sci. U.S.A. 122 (15) e2413503122 (2025). https://doi.org/10.1073/pnas.2413503122
Ribes, S. Qasmi, N. P. Gillett, Making climate projections conditional on historical observations. Sci Adv 7, eabc0671 (2021). https://doi.org/10.1126/sciadv.abc0671
Intergovernmental Panel on Climate Change (IPCC), Future Global Climate: Scenario-based Projections and Near-term Information in Climate Change 2021—The Physical Science Basis: Working Group I Contribution to the Sixth Assessment Report of the Intergovernmental Panel on Climate Change (Cambridge University Press, 2023), pp. 553–672. https://doi.org/10.1017/9781009157896
Gabriele Accarino and Francesco Immorlano are currently postdoctoral fellows at Learning the Earth with Artificial Intelligence & Physics (LEAP).