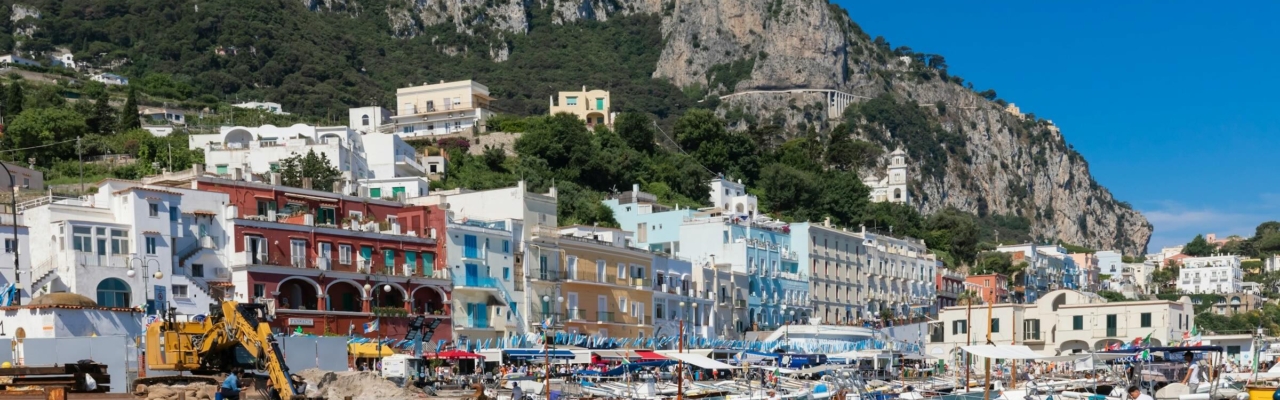
A novel application of machine learning to predict marine heatwaves in the Mediterranean Sea could help predict their impacts on marine ecosystems, fisheries, and coastal communities. New research, led by CMCC, shows how advanced data-driven techniques can enhance oceanographic predictions, potentially offering faster and more efficient forecasting tools compared to dynamical models.
Marine heatwaves (MHWs) have significant social and ecological impacts. Therefore, the prediction of these extreme events is essential to prevent and mitigate their negative consequences and provide valuable information to decision-makers about the nature and magnitude of specific MHW-related risks.
In a recent study, machine learning (ML) techniques are applied to predict sea surface temperature (SST) time series and marine heatwaves in 16 regions of the Mediterranean Sea. The study finds that ML techniques, such as Convolutional Neural Networks (CNN), Long Short-Term Memory (LSTM) networks, and Random Forest (RF) models, can predict sea surface temperature (SST) and marine heatwave (MHW) occurrences with reasonable accuracy.
For instance, the LSTM model achieved a positive score for one-day forecasts in the Western and Central Mediterranean and the ML methods performed with minimum errors in SST predictions were found to increase with forecast lead time but remained within acceptable limits for short-term predictions. Notably, the results from these ML models are comparable to those achieved by traditional dynamical models, indicating that ML can be a viable alternative for forecasting MHWs.
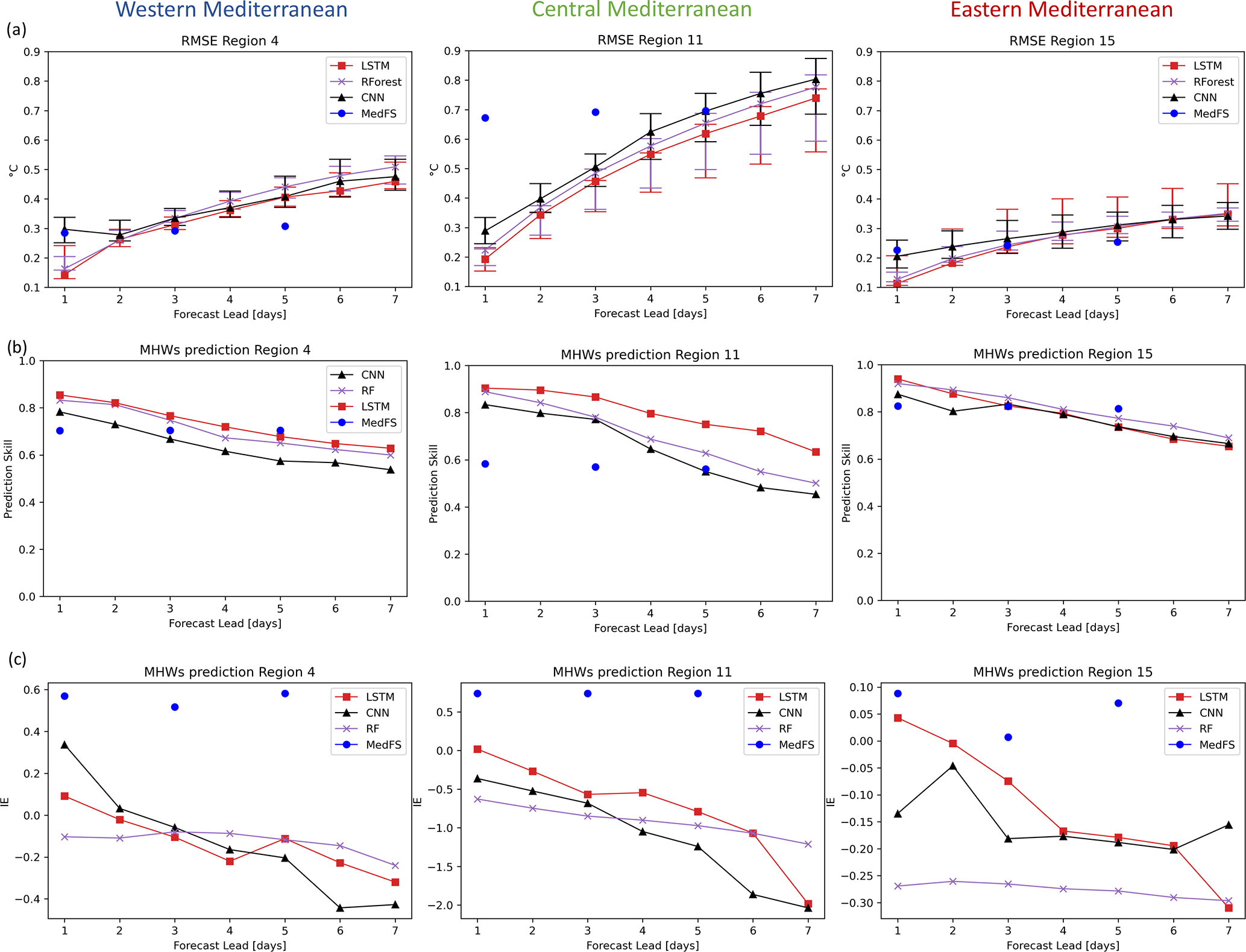
ML network performance for the SST daily predictions versus MedFS performance. Source: Bonino et al, 2024
“This represents a proof of concept in using ML to predict marine heatwaves. It is a starting point, demonstrating the potential of these techniques,” says lead author and CMCC researcher Giulia Bonino. “However, much more work needs to be done to refine these methods and expand their applications. In this field, studies applying artificial intelligence are in a nascent phase, and I am excited to see how they will evolve and contribute to our understanding of climate change impacts on marine environments.”
The CMCC played a key role in developing the methodological framework by performing experiments and interpreting results, as well as providing computational resources. This has helped provide valuable innovation in the integration of ML methods for short-term forecasting of SST and MHW occurrences, a topic which has not been extensively covered in existing literature, revealing that the use of advanced data-driven techniques to enhance oceanographic predictions has the potential to offer faster and more efficient forecasting tools.
More information:
Bonino, G., Galimberti, G., Masina, S., McAdam, R., and Clementi, E.: Machine learning methods to predict sea surface temperature and marine heatwave occurrence: a case study of the Mediterranean Sea, Ocean Sci., 20, 417–432, https://doi.org/10.5194/os-20-417-2024, 2024.