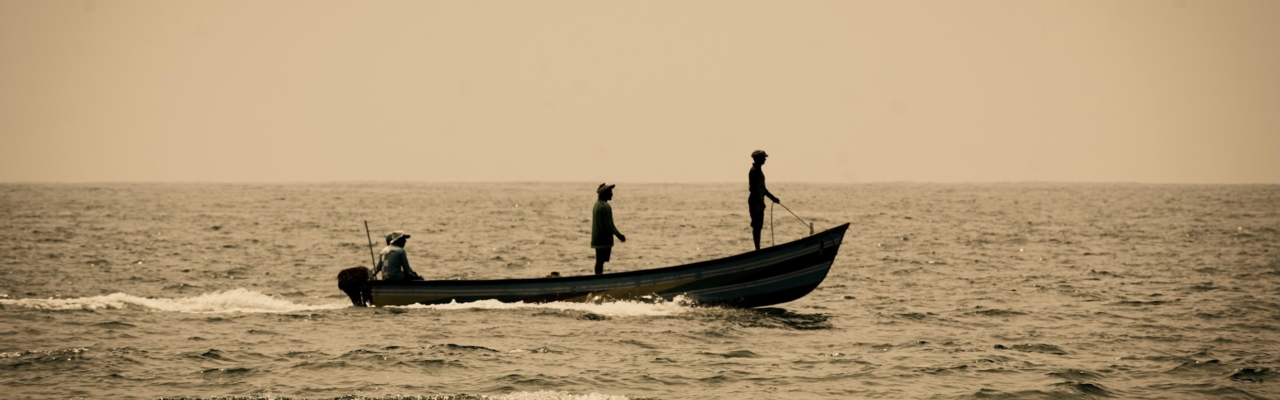
New research led by CMCC researcher Marie-Lou Bachèlery, harnesses the power of artificial intelligence (AI) to predict extreme Atlantic Niño and Benguela Niño events up to 3 months in advance. This offers an unprecedented opportunity to safeguard marine ecosystems and local economies, whilst also laying the groundwork for future applications on other hard-to-predict phenomena worldwide.
The Tropical Atlantic is home to some of the world’s richest marine ecosystems, including the Angola-Benguela Upwelling System along the west African coast. This unique region characterized by strong upwelling dynamics, supports a diverse marine ecosystem, and sustains the livelihoods of local fishing communities, as well as impacting those of distant nations.
However, these ecosystems face mounting challenges due to climate extremes like Atlantic Niño and Benguela Niño events – abnormal warming or cooling of ocean waters that disrupt marine life, fisheries productivity, and rainfall patterns.
“Overfishing alone cannot account for the fluctuations we are seeing in fish stocks. Climate extremes also play a crucial role, and predicting them has become a major scientific challenge,” says CMCC researcher Marie-Lou Bachèlery, lead author of Predicting Atlantic and Benguela Niño Events with Deep Learning, published in Science Advances.
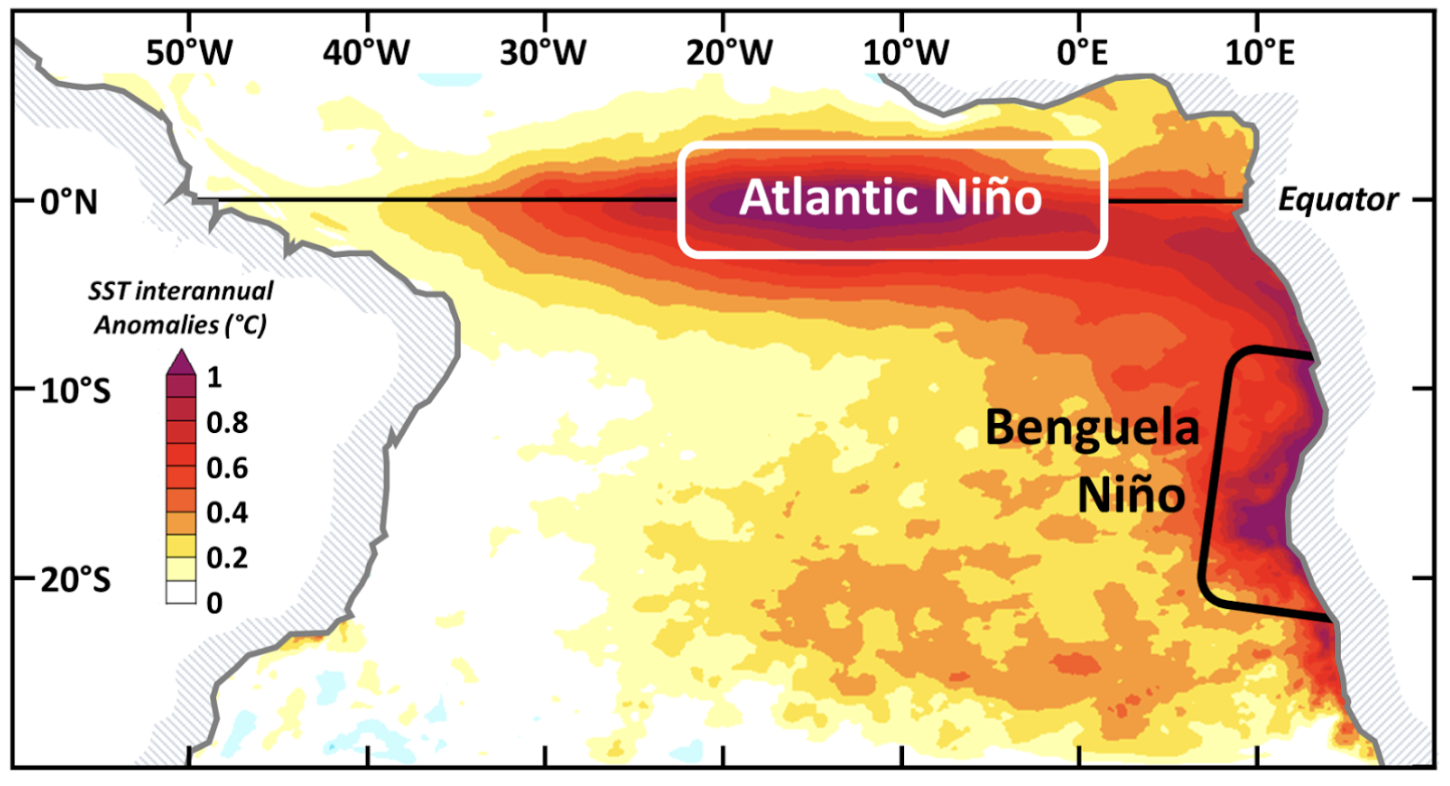
Warming hotspots in the tropical Atlantic: Atlantic and Benguela Niño regions highlighted by sea surface temperature anomalies.
For regions that depend on the ocean, early warning of such events could make a considerable difference, revolutionizing the way marine ecosystems are managed and securing the long-term sustainability of their resources. To address this challenge, the paper explores the potential of innovative techniques such as AI and deep learning algorithms.
The study takes a significant step forward in providing more accurate and reliable forecasts, challenging long-held beliefs about the unpredictability of tropical Atlantic climate events and also laying the groundwork for applying cutting-edge technology to other hard-to-predict phenomena worldwide.
This involved developing a convolutional neural network (CNN), a type of deep learning algorithm trained on 90 years of historical ocean temperature data. The AI model demonstrated remarkable accuracy, predicting these extreme events up to 3–4 months ahead – with even 5 months for peak-season occurrences. This level of advance warning surpasses traditional climate models, which often struggle to capture the complex interactions between ocean and atmosphere in the tropical Atlantic.
“Our deep learning model picked up on subtle precursors that lead up to these warming events, such as slow-moving ocean waves,” said Bachèlery. “By learning directly from data, the AI models overcame some of the recurring errors, such as biases and erroneous variability, in global climate models.”
One highlight was the accurate early prediction of the strong 2021 Atlantic and Benguela Niño events – 4 months before they occurred –while traditional systems missed them entirely.
“This is a significant innovation: we introduced cutting-edge AI to climate science in a way that not only improves predictions but also offers a new perspective on understanding extreme events. It’s a template that could be applied to other hard-to-predict climate phenomena in the future,” says Bachèlery.
One of the next steps includes the development of a web platform for sharing forecasts, so that people on the ground – such as fishers, marine resource managers, and coastal planners – can access early warnings of these events.
“It’s vital that our forecasts align with the needs of those who depend on marine ecosystems,” says Bachèlery. “By involving local stakeholders and tailoring information for them, we hope these predictions will improve decision making processes and prepare communities for the impacts of Atlantic and Benguela Niños. Seeing our research move from academia into a tool that benefits society is incredibly rewarding.”
At CMCC Bachèlery plans to expand on the study – which was conducted during a Marie Curie fellowship at the University of Bergen in Norway, in collaboration with NERSC, University of Cambridge, and LEGOS in France – by continuing to improve the model and applying it to forecast other important variables like oxygen levels and fishery productivity.
For more information:
Marie-Lou Bachèlery et al.,Predicting Atlantic and Benguela Niño events with deep learning.Sci. Adv.11, eads5185(2025). DOI:10.1126/sciadv.ads5185